News
Inclusive AI: Representation of Age, Gender, and Race in Accessibility Datasets
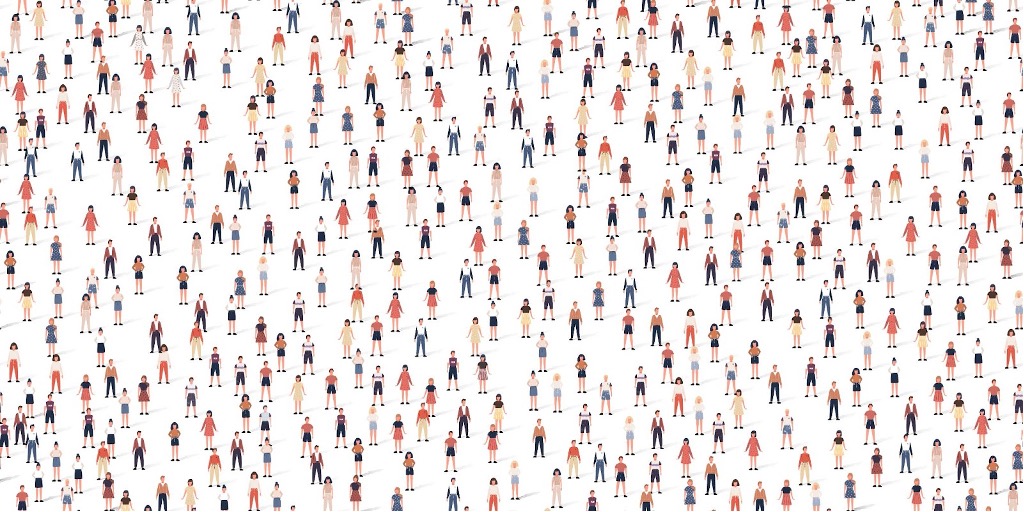
Image by svstudioart on Freepik
More and more technologies that we use in our everyday lives are supported by artificial intelligence (AI). AI-infused technologies help us unlock our mobile phones, power our digital assistants, monitor health conditions, detect financial fraud, and navigate the best travel route. Although AI affords numerous conveniences and efficiencies to users, it can lead to discriminatory outcomes for historically marginalized groups who are often underrepresented as contributors to datasets used to train and evaluate the machine learning models behind AI. It is critical that these issues of fairness and inclusion are addressed by researchers and developers so that the benefits of AI can be equally shared and the potential negative consequences—e.g., less responsive health care or discriminatory surveillance—are mitigated.
To respond to these concerns, researchers from the University of Maryland’s College of Information Studies and Department of Computer Science analyzed a collection of accessibility datasets to understand the representation and reporting of their contributors’ demographic information, specifically gender, age, and race and ethnicity. The results of this analysis are reported in “Data Representativeness in Accessibility Datasets: A Meta-Analysis,” a paper by Rie Kamikubo, Lining Wang, Crystal Marte, Amnah Mahmood, and Dr. Hernisa Kacorri (core faculty and principal investigator at the Trace R&D Center), to be presented at ASSETS ’22, the 24th International ACM SIGACCESS Conference on Computers and Accessibility in Athens, Greece, in October, 2022.
The team used the 190 datasets sourced from people with disabilities and older adults that can be found in IncluSet, a data-surfacing repository launched by Kacorri’s team in 2019. The purpose of IncluSet is related to similar issues of representation; its focus is on making datasets sourced from people with disabilities and older adults more visible to researchers and developers so that machine learning models can be trained and AI-systems benchmarked with data from a wider range of users. The current study goes one step further by exploring the intersectionality of marginalized identities represented and reported in the datasets. Disability communities of focus represented in the collection include autism, cognitive, developmental, health, hearing, language, learning, mobility, speech, and vision. Data types captured by these datasets include audio, video, text, motion, image, logs, and sensing data.
The researchers found that while there is diversity of ages represented in the data (though less so in the autism, developmental, and learning disability communities), there are significant gaps in gender and race and ethnicity representation. The underlying reasons for these gaps are complex. There are structural forces at play; for example, there are fewer women and girls diagnosed with autism, a consequence of the prevailing diagnostic criteria which make identification of men and boys more likely. There are inconsistent norms for reporting data with respect to categorizations and documentation standards that reflect social and cultural norms and biases. For example, most datasets that documented gender included only binary gender information. Further, some datasets included inferences about the gender of contributors based on visual inspection of video content or the contributors’ profiles. With respect to race and ethnicity, there are significant reporting differences in datasets originating in different countries or regions, where meaningful categories of race and ethnicity may vary.
The paper explores a variety of implications arising from this work and points to the importance of researchers in accessibility taking considerable care as they work to include marginalized communities within their systems. Unintended consequences are a major concern. For example, there is the possibility that datasets collected to reduce bias in AI-infused systems could actually be used to detect undisclosed disabilities in users, leading to further risks of discrimination. One conclusion is that it’s important that data contributors are meaningfully engaged in participatory approaches to data collection, maintenance, sharing, and interpretation so that their values can be reflected in the process.
Some of the possible future research directions identified by Kacorri and her team include:
- Exploring perceptions of people with disabilities as potential contributors to AI datasets, to have a better understanding of their concerns such as risks to privacy or surveillance which may outweigh the benefits of representativeness.
- Examining the sociocultural contexts in which datasets are produced (e.g., from the HCI vs. medical research community) to challenge other social and structural biases that may be found within current data practices.
- Evaluate the impact of datasets using different metrics including frequency of citations, which and how many ML models that are used to train or benchmark, and whether they are used in academic research or by industry in development of commercial products.
- Looking at representativeness beyond accessibility datasets and considering the impact of accessibility as a means of diversifying representation in the broader AI community.
The overarching concern of this work is the greater inclusion of marginalized communities in AI-infused systems. To explore more work by Dr. Kacorri and her team on Inclusive AI, visit the project page for this research. This work is funded by the Inclusive Information and Communications Technology RERC (90REGE0008) from the National Institute on Disability, Independent Living, and Rehabilitation Research (NIDILRR), Administration for Community Living (ACL), Department of Health and Human Services (HHS). Learn more about the work of the Inclusive ICT RERC.
—-
Kamikubo, R., Wang, L., Marte, C., Mahmood, A., & Kacorri, H. (2022). Data representativeness in accessibility datasets: A metaanalysis. In ASSETS ’22: The 24th International ACM SIGACCESS Conference on Computers and Accessibility (pp. 1-15). No. New York: ACM. https://arxiv.org/abs/2207.08037